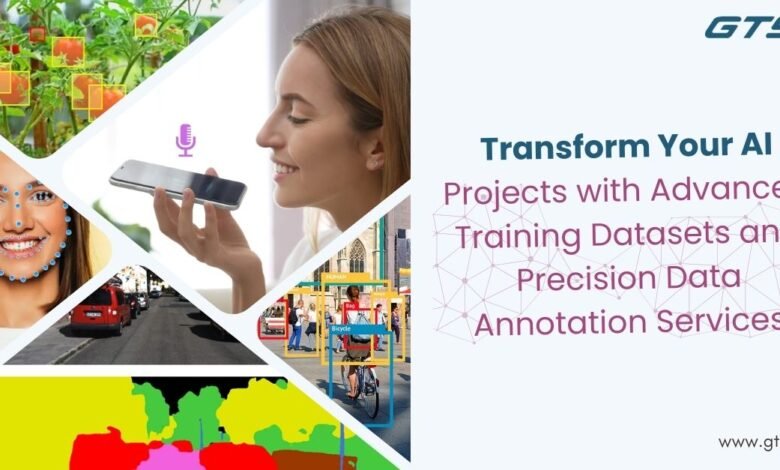
Introduction
In the era of big data and machine learning, audio datasets play a pivotal role in shaping technologies that require sound understanding and interaction. These datasets are the backbone of numerous applications, from voice-activated assistants to automated music recommendation systems. But what exactly are audio datasets, and why are they so crucial? This blog dives deep into the world of audio data, exploring its types, applications, and the challenges involved in its creation and use.
Understanding Audio Datasets
Audio datasets consist of sound recordings and their corresponding annotations. These sounds can range from human speech and ambient noises to musical compositions. The data is often stored in digital formats like WAV or MP3 and is meticulously labeled to train machine learning models effectively. For instance, a dataset might tag parts of a recording with words spoken or identify various instruments in a song.
Types of Audio Datasets
Audio datasets vary widely, each serving different purposes:
- Speech Datasets: Essential for developing speech recognition systems, these datasets help in training algorithms to understand and generate human speech. Examples include datasets used by developers to enhance the responsiveness of virtual assistants like Siri and Alexa.
- Environmental Sound Datasets: These datasets encompass a range of sounds from our surroundings, such as traffic noise, rain, or office ambiance. They are crucial for applications like urban planning where sound level monitoring is needed.
- Music Datasets: Used in the entertainment and media industry, these datasets assist in music classification, recommendation, and even composition, fostering innovations in how we discover and enjoy music.
- Multi-purpose Datasets: Some datasets are designed to be versatile, containing a mix of sounds which can be used to train more robust and flexible models.
Applications of Audio Datasets
The applications of audio datasets are vast and varied:
- Machine Learning and AI: These technologies stand at the forefront, using audio datasets to train algorithms that can recognize, interpret, and generate sound-based data.
- Academia: Researchers utilize audio data to advance knowledge in fields such as linguistics, acoustics, and psychology.
- Industry Applications: From automotive systems that respond to voice commands to healthcare devices that monitor and analyze patient sounds, audio datasets are increasingly crucial.
Challenges in Audio Data Collection and Processing
Collecting and processing audio data presents several challenges:
- Privacy and Legality: Recording audio often involves navigating complex privacy laws and ethical considerations, particularly with speech data.
- Technical Challenges: Ensuring the audio quality and variability needed for robust datasets can be technically demanding and expensive.
- Annotation and Labeling: Audio data requires precise and often labor-intensive labeling that can significantly increase the time and cost of dataset preparation.
Creating an Audio Dataset
Creating an audio dataset involves several key steps:
- Planning: Define the scope and type of sounds to be included.
- Recording and Collecting: Gather audio using devices suited to the task while ensuring a diverse and comprehensive collection.
- Annotation: Label the collected sounds accurately, a step that might require expert knowledge, especially for complex sounds or languages.
- Storage and Accessibility: Store the data in a format that is easily accessible and widely compatible for various uses.
Notable Audio Datasets
Some well-known audio datasets include:
- LibriSpeech: Widely used in speech recognition research, it contains thousands of hours of spoken English from audiobooks.
- UrbanSound8K: A collection of urban sounds from New York City, useful in developing applications that identify urban noises.
- ESC-50: Comprising 50 classes of environmental sounds, this dataset aids in building more accurate environmental sound classification systems.
- Google’s AudioSet: A large-scale dataset consisting of millions of YouTube video soundtracks annotated to provide a balanced audio dataset.
Ethical Considerations in Audio Data Usage
As the use of audio datasets expands, ethical considerations become increasingly important. Here are a few key aspects:
- Consent and Privacy: Ensuring that all audio recordings are obtained with explicit consent is crucial. For instance, voice recordings that are used to train speech recognition systems must adhere to strict privacy regulations to protect personal information.
- Bias and Fairness: Audio datasets, like any data used in AI training, can contain biases which may lead to unfair outcomes in AI applications. Ensuring that datasets are diverse and representative of different demographics is essential to mitigate this risk.
- Transparency: Companies and researchers should be transparent about how audio data is used, particularly in applications that directly affect people, such as in hiring processes or law enforcement.
Future Trends in Audio Data
The future of audio datasets is likely to be shaped by advances in technology and changes in societal norms and regulations:
- Increased Use of Synthetic Data: To overcome challenges related to privacy and diversity, more organizations are turning to synthetic audio data. These are artificially created sounds and voices that can help train robust models without the ethical and legal issues associated with real human data.
- Advancements in Audio Processing Technologies: Innovations in digital signal processing and AI are making it possible to extract more meaningful information from audio data than ever before. This could lead to more sophisticated applications in health diagnostics, environmental monitoring, and interactive entertainment.
- Regulatory Developments: As the use of audio data grows, so does regulatory interest. Future trends will likely include stricter regulations on how audio data can be collect, use, and share, particularly in sensitive areas like healthcare and public surveillance.
- Integration with Other Data Types: Audio data is increasingly being combine. With other types of data (like visual and textual data) to build more comprehensive AI models. This multimodal approach is enhancing capabilities in areas such as multimedia content analysis and multimodal learning systems.
The Future of Audio Datasets
As technology evolves, so too does the role of audio datasets. Advances in AI and machine learning continue to push the boundaries. With new applications and improvements in dataset creation, processing, and utilization appearing on the horizon.
Conclusion
Audio datasets are more than just collections of sounds. They are the foundations upon which many of the cutting-edge technologies of our time are built. As we continue to explore and innovate in this area. The potential for new applications and improvements in sound-based technology seems almost limitless.