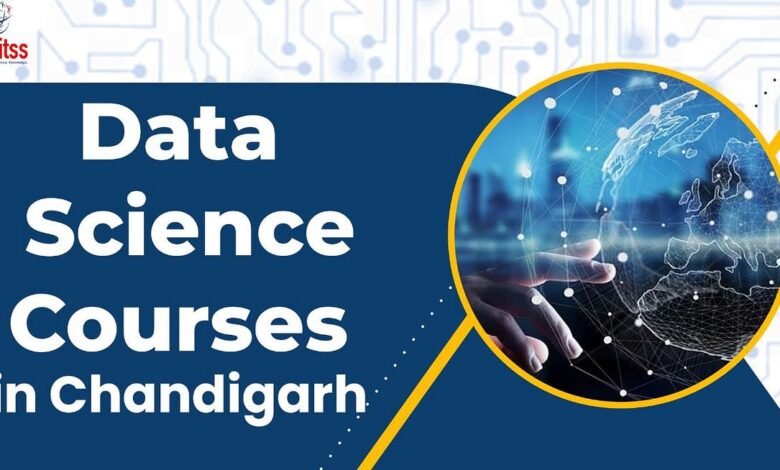
What is the eligibility criteria for a data science course?
Table of Contents:
-
- Introduction
- Understanding Predictive Analytics
- The Importance of Predictive Analytics
- Enhancing Decision-Making Processes
- Anticipating Future Trends and Patterns
- Mitigating Risks and Identifying Opportunities
- Key Components of Predictive Analytics
- Data Collection and Preparation
- Modeling Techniques
- Evaluation and Validation
- Industries Benefiting from Predictive Analytics
- Healthcare
- Finance
- Retail
- Manufacturing
- Challenges and Considerations
- Data Quality and Integration
- Privacy and Ethical Concerns
- Model Interpretability
- Conclusion: Embracing the Power of Predictive Analytics
 Introduction
In an era where data reigns supreme, organizations across industries are increasingly turning to predictive analytics to gain valuable insights and stay ahead of the curve. Predictive analytics offers a powerful toolset that enables businesses to forecast future outcomes, identify trends, and make informed decisions. In this blog post, we will delve into the significance of predictive analytics and explore why it has become indispensable in today’s data-driven world. Additionally, we’ll address the query: What is the eligibility criteria for a data science course?
Understanding Predictive Analytics
Predictive analytics involves the use of statistical algorithms and machine learning techniques to analyze historical data and predict future outcomes. By uncovering patterns and relationships within vast datasets, predictive analytics empowers organizations to anticipate trends and make proactive decisions. Whether it’s forecasting customer behavior, predicting market demand, or identifying potential risks, predictive analytics offers invaluable insights that drive business success.
The Importance of Predictive Analytics
Enhancing DecisionMaking Processes
One of the primary benefits of predictive analytics is its ability to enhance decision making processes. By leveraging predictive models, organizations can make data driven decisions with greater confidence and accuracy. Whether it’s optimizing marketing campaigns, identifying highvalue customers, or streamlining operations, predictive analytics provides actionable insights that drive strategic decision making.
Anticipating Future Trends and Patterns
In today’s rapidly evolving business landscape, the ability to anticipate future trends and patterns is crucial for staying competitive. Predictive analytics enables organizations to identify emerging trends, anticipate shifts in consumer behavior, and adapt their strategies accordingly. By analyzing historical data and extrapolating future outcomes, predictive analytics empowers businesses to stay ahead of the curve and capitalize on new opportunities.
Mitigating Risks and Identifying Opportunities
Risk management is another area where predictive analytics plays a pivotal role. By analyzing historical data and identifying potential risks, organizations can proactively mitigate threats and safeguard their interests. Whether it’s detecting fraudulent activities, assessing credit risks, or predicting equipment failures, predictive analytics helps organizations identify and address risks before they escalate. Additionally, predictive analytics can uncover untapped opportunities and help businesses capitalize on new market segments or product offerings.
Key Components of Predictive Analytics
Data Collection and Preparation
The first step in predictive analytics is gathering and preparing the data. This involves collecting relevant datasets from various sources, cleaning and preprocessing the data to remove noise and inconsistencies, and transforming the data into a format suitable for analysis.
Modeling Techniques
Once the data is prepared, the next step is to select and apply appropriate modeling techniques. This may include statistical methods such as regression analysis, machine learning algorithms such as decision trees or neural networks, or a combination of both. The choice of modeling technique depends on the nature of the data and the specific problem being addressed.
Evaluation and Validation
After developing predictive models, it’s essential to evaluate their performance and validate their accuracy. This involves testing the models against historical data to assess their predictive power and ensure they generalize well to new data. By rigorously evaluating and validating predictive models, organizations can have confidence in their ability to make accurate predictions.
Industries Benefiting from Predictive Analytics
Healthcare
In the healthcare industry, predictive analytics is revolutionizing patient care and clinical decisionmaking. From predicting disease outbreaks to identifying highrisk patients, predictive analytics helps healthcare providers deliver more personalized and effective treatments while reducing costs and improving outcomes.
Finance
In the finance sector, predictive analytics is used for risk management, fraud detection, and investment forecasting. By analyzing market data and customer behavior, financial institutions can identify potential risks, detect fraudulent activities, and make informed investment decisions.
Retail
In the retail industry, predictive analytics is employed for demand forecasting, inventory optimization, and customer segmentation. By analyzing sales data and customer preferences, retailers can anticipate demand, optimize inventory levels, and deliver personalized shopping experiences that drive sales and customer loyalty.
Challenges and Considerations
Data Quality and Integration
One of the key challenges in predictive analytics is ensuring the quality and integration of data. Poor data quality or incomplete data can lead to inaccurate predictions and unreliable insights. Organizations must invest in data quality assurance processes and integrate data from disparate sources to ensure the accuracy and reliability of predictive models.
Privacy and Ethical Concerns
Another challenge is navigating privacy and ethical concerns associated with predictive analytics. The use of personal data for predictive modeling raises important ethical considerations around consent, transparency, and fairness. Organizations must adhere to strict data privacy regulations and ethical guidelines to ensure the responsible use of predictive analytics.
Model Interpretability
Finally, model interpretability is a critical consideration in predictive analytics. Complex machine learning algorithms may produce accurate predictions, but they can be challenging to interpret and explain. Organizations must prioritize the interpretability of predictive models to gain insights into the factors driving predictions and build trust among stakeholders.
Conclusion: Embracing the Power of Predictive Analytics
In conclusion, predictive analytics is a powerful tool that offers organizations the ability to forecast future outcomes, identify trends, and make informed decisions. By leveraging predictive analytics, businesses can enhance decision-making processes, anticipate future trends, and mitigate risks. Despite the challenges and considerations involved, the potential benefits of predictive analytics are vast, making it an essential capability in today’s data-driven world. As organizations continue to harness the power of predictive analytics, they will gain a competitive edge and drive innovation in their respective industries.
Additionally, individuals interested in pursuing a career in data science may wonder, the eligibility criteria for a data science course. This question is crucial as it varies depending on the institution and the specific course. Typically, candidates are required to have a strong background in mathematics, statistics, computer science, or related fields. Proficiency in programming languages such as Python, R, or SQL is often necessary. Moreover, some courses may require prior experience in data analysis or related fields. Checking the specific eligibility requirements of the desired data science course is essential for prospective students to ensure they meet the criteria before applying.