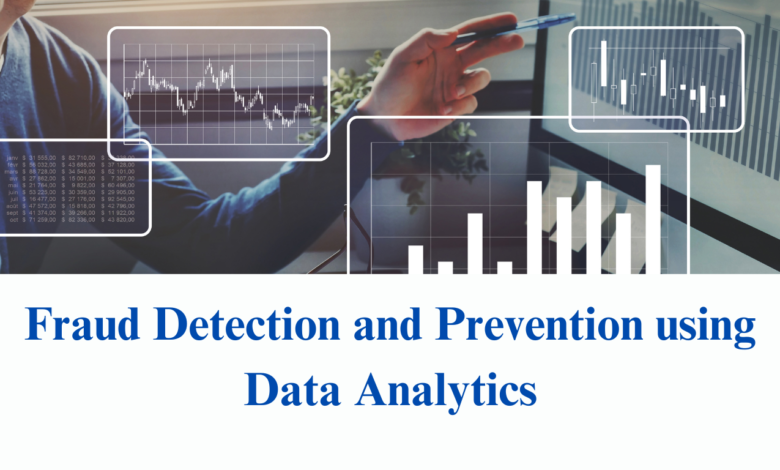
Introduction
In an era defined by digital transactions and technological advancements, the specter of fraud looms large, necessitating robust measures for detection and prevention. This introduction unravels the imperative of leveraging Data Analytics in the realm of fraud detection, an indispensable approach to safeguarding businesses and individuals from deceptive practices.
Fraud, in its multifaceted forms, poses a constant threat to the integrity of financial transactions, digital platforms, and sensitive information. The proactive utilization of Data Analytics becomes pivotal in this context, offering a dynamic toolkit to unravel patterns, anomalies, and irregularities indicative of fraudulent activities. As we navigate the complex landscape of fraud, the synergy between data-driven insights and preventive strategies emerges as a linchpin, steering us towards a future where adaptive analytics stand guard against the ever-evolving tactics of those seeking to exploit vulnerabilities in our interconnected systems.
What are the Types of Fraud?
Understanding the landscape of fraud requires a nuanced exploration of its various manifestations. Fraud comes in diverse forms, from identity theft and payment card fraud to insider threats and cyber attacks. By classifying these deceptive practices, organizations can tailor their fraud detection strategies to specific threats.
Identity theft involves the unauthorized acquisition of personal information for fraudulent purposes, while payment card fraud targets financial transactions through unauthorized use of credit or debit cards. Insider threats, on the other hand, involve deceitful actions by individuals within an organization for personal gain.
Data Sources for Fraud Detection
Effective fraud detection hinges on the richness of data sources available for analysis. Diverse streams of data, including transaction records, user profiles, and system logs, serve as valuable reservoirs for uncovering anomalies. By harnessing structured and unstructured data, organizations can paint a comprehensive picture of normal and abnormal behavior.
Transaction data provides insights into patterns of spending and financial activities, while user profiles offer information about individual behaviors and historical interactions. System logs capture the footprints of digital activities, helping to identify suspicious access or modifications. The synergy of these data sources empowers Data Analytics to sift through immense datasets, revealing hidden patterns indicative of fraudulent activities.
Predictive Analytics in Fraud Detection
Predictive analytics plays a pivotal role in fraud detection by employing advanced modeling techniques to anticipate and prevent deceptive practices. By analyzing historical data and identifying patterns indicative of fraudulent behavior, predictive analytics becomes a proactive shield against emerging threats.
Machine learning algorithms are instrumental in predictive analytics for fraud detection. These algorithms learn from historical fraud cases and continuously adapt to new patterns, enabling organizations to stay ahead of evolving tactics. Predictive models assess the likelihood of a transaction or activity being fraudulent based on a combination of factors, such as transaction amount, location, and user behavior.
These models assign risk scores to transactions or activities, flagging those with higher likelihoods of fraud for further investigation. This enables organizations to prioritize their resources and focus on the most potentially fraudulent cases. The dynamic nature of predictive analytics ensures that fraud detection strategies remain adaptive in the face of ever-changing fraud techniques.
Real-time Monitoring and Alerts
In the fast-paced landscape of digital transactions, real-time monitoring and alerts are crucial components of effective fraud detection. Real-time analytics systems continuously analyze incoming data and transactions as they occur, enabling instant detection of suspicious activities.
By implementing real-time monitoring, organizations can swiftly respond to potential fraud, mitigating risks and minimizing financial losses. Automated alerts are triggered when anomalies or deviations from normal patterns are identified, prompting immediate action. This timely response is essential for preventing further fraudulent activities and safeguarding both businesses and consumers.
Real-time monitoring is icularly vital in industries such as finance, where transactions occur rapidly and fraudsters exploit vulnerabilities in milliseconds. The integration of data analytics in real-time systems enhances the speed and accuracy of fraud detection, providing a proactive defense against unauthorized transactions and illicit activities.
In essence, the combination of predictive analytics and real-time monitoring forms a dynamic duo in the fight against fraud. By leveraging the power of data analytics to predict and identify potential fraud in real-time, organizations can fortify their defenses and create a robust fraud detection infrastructure. This synergy ensures that fraudulent activities are not only detected promptly but also preemptively thwarted before they can inflict significant damage.
Machine Learning in Fraud Prevention
Machine learning stands as a cornerstone in the realm of fraud prevention, offering adaptive strategies to combat the ever-evolving landscape of fraudulent activities. Through the continuous learning and refinement of algorithms, machine learning models excel at detecting anomalies and patterns indicative of fraud.
One notable application is anomaly detection, where machine learning algorithms establish a baseline of normal behavior and flag deviations as potential fraud. These models autonomously adapt to changes in patterns, enabling them to identify novel and sophisticated fraud schemes that might elude traditional rule-based systems.
Machine learning also plays a crucial role in adaptive authentication. By analyzing user behavior, such as keystroke dynamics or device usage patterns, machine learning models create unique user profiles. Any deviation from these profiles triggers alerts, signaling potential unauthorized access or fraudulent activities.
Additionally, machine learning facilitates the creation of dynamic risk scores for transactions. By considering multiple factors, such as transaction amount, location, and historical behavior, machine learning models assess the risk associated with each transaction in real-time. Transactions with elevated risk scores can then be subjected to additional scrutiny or flagged for further investigation.
You Should Also Read:-
Python Training : Empowering Developers with Python Skills
Analyzing Data, Shaping Futures: The Realm of Data Analytics
Behavioral Analytics
Behavioral analytics emerges as a powerful tool in fraud prevention, focusing on understanding patterns for individualized detection. By analyzing the digital behavior of users, organizations can establish baselines for normal behavior and identify deviations that may indicate fraudulent activities.
Behavioral analytics considers various factors, including login times, transaction history, and navigation patterns. Machine learning algorithms analyze these behavioral patterns to create unique profiles for users. Any aberration from established patterns prompts alerts, allowing organizations to investigate and take preventive measures promptly.
This approach is icularly effective in identifying account takeover attempts and insider threats. By monitoring for unusual patterns, such as an account accessing sensitive information from an unfamiliar location or at an unusual time, behavioral analytics aids in detecting potentially malicious activities early in the process.
Moreover, behavioral analytics enhances user-centric fraud prevention. Instead of solely relying on static rules, this approach considers the evolving behavior of users, adapting to changes in their digital interactions over time. This ensures a more personalized and effective fraud prevention strategy that evolves with the dynamic nature of user behavior.
In conclusion, the integration of machine learning and behavioral analytics represents a paradigm shift in fraud prevention. These approaches not only enhance the accuracy and efficiency of fraud detection but also enable organizations to proactively respond to emerging threats. By leveraging the capabilities of machine learning and understanding the nuances of user behavior, organizations can create a robust defense against the constantly evolving landscape of fraudulent activities with the help of Data Analytics Course in Delhi, Patna, Ludhiana, Jaipur, etc.
Conclusion
In the relentless pursuit of combating fraud, the marriage of Data Analytics, machine learning, and behavioral analytics emerges as an indomitable force. Consequently, as we navigate the multifaceted landscape of fraud detection and prevention, it becomes evident that the strategic application of data-driven insights and adaptive technologies is paramount in safeguarding financial transactions and digital ecosystems. The synergy of predictive analytics, real-time monitoring, machine learning, and behavioral analytics fortifies organizations against evolving fraud schemes. These proactive measures not only detect fraud promptly but also preemptively thwart potential threats, preserving the integrity of businesses and securing sensitive information.
For those aspiring to delve into this dynamic field, consider exploring an Offline Data Science Certification Course in Delhi. These educational pathways equip professionals with the skills necessary to navigate the intricate world of fraud detection and prevention, fostering a new generation of experts dedicated to upholding the integrity of digital transactions in an interconnected era.